

Appel à projets
L'appel à projets pédagogiques et numériques est ouvert jusqu'au 27 mai 2024.
Le guide et le document de réponse sont disponibles sur la page dédiée ci-dessous.

Intelligence artificielle
L’université Paris 1 Panthéon-Sorbonne encourage les espaces de réflexion, d’expérimentation et de partage de pratiques
Présentation du SUN
Le SUN accompagne les enseignements
Projet

Peut-on réviser les cours avec Archéo-Bot ?
Un agent conversationnel intelligent pour approfondir les notions d'archéologie... Composante : Département Histoire de l'art...
Regard sur

Retour sur la Rencontre Webinaire "L'IA et nous"
Enseigner avec l'intelligence artificielle : Chat bot, QCM, tâches administratives, premiers retours d'expérimentations...
Projet

Modules for Becoming Autonomous in the Library
Summary version in English Department: SCD (University Library Department)
Project Leaders: Anne GUICHARD-CAZENAVE, Imelda LEMOING...
Atelier
Image de couverture

Atelier
Image de couverture

Le jeudi 6 juin 2024
10h00
12h00
Définir et décrire le programme d'un cours
Atelier
Image de couverture

Le mardi 11 juin 2024
10h00
12h00
Mettre en place une formation hybride ou à distance
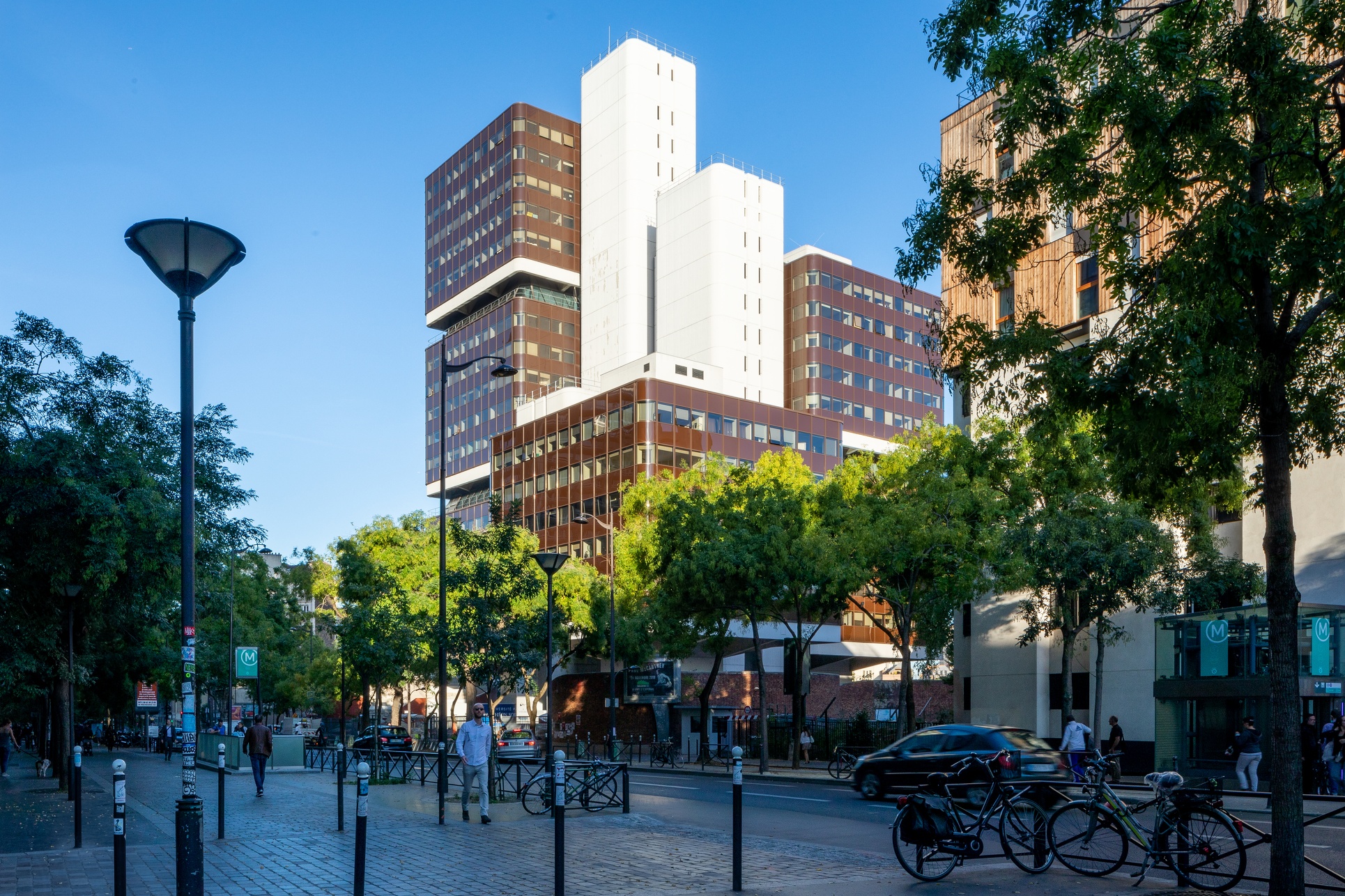
Centre Pierre Mendès France
31, rue Baudricourt 75013 Paris
Se rendre au centre Centre Pierre Mendès France en transports
Se rendre au centre Centre Pierre Mendès France à pied
Se rendre au centre Centre Pierre Mendès France à vélo
Se rendre au centre Centre Pierre Mendès France en voiture
Service des usages numériques
A 806